Introduction
The Center for News, Technology & Innovation (CNTI) hosted its inaugural event convening leaders in journalism, technology, policy and research for an evidence-based discussion about enabling the benefits and managing the harms of AI use in journalism, with a focus on critical definitional considerations when constructing policy.
Co-sponsored by and hosted at the Computer History Museum in Mountain View, California, the Oct. 13 event brought together legal and intellectual property experts from OpenAI, Google and Microsoft; leading journalists from The Associated Press, Axios, Brazil’s Nucleo Journalismo and Nigeria’s Premium Times; researchers in AI and intellectual property law from the University of Oxford, the University of Sussex, Research ICT Africa and Stanford; and technology policy and industry experts representing a range of organizations.
Participants
- Anna Bulakh, Respeecher
- Garance Burke, The Associated Press
- Craig Forman, NextNews Ventures
- Richard Gingras, Google
- Andres Guadamuz, University of Sussex
- Dan’l Lewin, Computer History Museum
- Megan Morrone, Axios
- Dapo Olorunyomi, Premium Times
- Matt Perault, Center on Technology Policy
- Ben Petrosky, Google
- Kim Polese, CrowdSmart
- Aimee Rinehart, The Associated Press
- Tom Rubin, OpenAI
- Marietje Schaake, Stanford Cyber
- Policy Center (moderator)
- Felix Simon, Oxford Internet Institute
- Krishna Sood, Microsoft
- Sérgio Spagnuolo, Núcleo Jornalismo
- Scott Timcke, Research ICT Africa
For more details, see the Appendix.
Among the questions considered were: How should policy define “artificial intelligence” in journalism, and what should fit into that bucket? How do we use language that plans for future technological changes? What are the important complexities related to copyright considerations around AI in journalism?
The productive session, held under a modified Chatham House Rule, sets the tone for the many convenings CNTI will hold in the months and years to come across a range of issues facing our digital news environment: using research as the foundation for practical, collaborative, solutions-oriented conversations among thoughtful leaders who don’t agree on all the elements, but who all care about finding solutions that safeguard an independent news media and access to fact-based news. As one participant put it, even just for AI: “We need 50, 100, of these.”
Key Agreed-Upon Principles:
- Better articulation, categorization and understanding of AI is essential for productive discussions.
- Whether a particular AI use is a benefit or harm to society depends on its context and degree of use, making specificity vital to effective policy.
- Even when policy is groundbreaking, it must also take into account how it relates to and builds on prior policy.
- One policy goal should be to address disparities in the uses and benefits of AI as a public good.
- Both inputs and outputs, at all stages of building and using AI, need to be considered thoroughly in policy development.
The day concluded with ideas for next steps, including taking stock of AI use cases in journalism, creating clear and consistent definitions of news and news publishers, and examining copyright laws to better understand how exactly they apply to AI use in news.
Stay tuned for CNTI’s second AI convening, which will consider oversight structures assigned to organizational and legislative policies around AI use in journalism.
1. BETTER ARTICULATION, CATEGORIZATION AND UNDERSTANDING OF AI IS ESSENTIAL FOR PRODUCTIVE DISCUSSIONS.
Cross-industry experts unanimously agreed on the need to work together to better define AI and articulate clear categories of use. This will enable a common understanding and allow for more productive conversations. Right now, that is not happening. As one participant remarked, “We jump into the pool … and we’re not even talking about the same thing.”
An overarching definition of AI: The participants chose not to spend their limited time together writing a precise definition of AI, but they shared definitions they have found to be useful starting points including those from:
- Council of the EU: “systems developed through machine learning approaches and logic- and knowledge-based approaches.”
- AP Stylebook’s AI chapter: separate definitions for “artificial intelligence,” “artificial general intelligence,” “generative AI,” “large language models” and “machine learning.”
- Melanie Mitchell: “Computational simulation of human capabilities in tightly defined areas, most commonly through the application of machine learning approaches, a subset of AI in which machines learn, e.g., from data or their own performance.”
Research backs up the need for, and lack of, clarity around AI.
A lack of conceptual clarity around AI, changing interpretations of what qualifies as AI and the use of AI as an umbrella term in practice and policy can make potential violations of law incredibly hard to identify and enforce. Recent legislative activity around AI, including the European Union’s AI Act has been criticized for offering a broad legal definition of “general purpose AI systems,” making it difficult to know what would be included or not included in this scope. Canada’s Bill C-27, similarly, does not define the “high-impact systems” it is regulating. It is important to proceed from shared understandings of the technology at issue and the harms policymakers hope to address. For example, attempting to write laws touching only “generative AI” (e.g., chatbots, LLMs) could inadvertently prohibit processes that underlie a range of technological tools, while applying requirements to broad or vaguely defined categories of technology could lead to legal uncertainty and over-regulation.
Within these definitions are several categories of use that must also be clearly differentiated and articulated. They include:
The scope of AI: AI is not new – in general nor in journalism – and represents a much broader set of technologies than simply generative AI (GAI) or large language models (LLMs). Nevertheless, one participant noted research finding many burgeoning AI newsroom policies narrowly define AI as GAI and LLMs, which “speaks to the challenges of grasping this set of technologies.”
The type of AI use: It is important to differentiate among the various types of AI use related to news. There are uses for news coverage and creation and, within that, questions around the degree of human vs. AI involvement in published content. There are uses that help with newsroom efficiency and cost savings, such as transcription, that don’t necessarily result in public-facing AI output. There are uses for news distribution and access, such as translation. At least one participant remarked on the tendency, when thinking about LLMs, to limit the scope of its definition to text-based GAI rather than comprehensively conceptualize GAI to include other formats such as XtownLA, which uses AI models for reporting on public hearings.
Who the user is: It is critical to specify who the user is at each point in the process of AI use so that appropriate responsibility, and perhaps liability, can be attributed. Is it newsrooms? Members of the public? Technology or third-party companies? Governments? This articulation is often missed.
The part of the AI system being accessed: A few participants talked about the importance of understanding and differentiating among the different levels of AI systems. The first level is, for example, the LLM being built. The second level is the API or corpus (e.g., all data being used as inputs) of what is in the model or further steps such as reinforcement learning through human feedback (RLHF). Third is the application use level, such as ChatGPT, in which humans do not actually interact with the model itself. Each of these, as one participant noted, should have different policy considerations.
Another pitfall in current discussions occurs when we don’t take time to articulate what is neither a part of AI nor tied directly to its use. This is particularly important when it comes to guarding against harms. Participants articulated the need to distinguish between which issues are attributed to the internet and social media age in general and which issues are linked specifically to AI technologies.
Better understanding of these definitions and categorizations can also help build trust, which several participants named as critical for positive outcomes from AI use and policy development. One participant asked, “How do we generate trust around something that is complicated, complex, new and continuously changing?” Another added, “Trust is still really important in how we integrate novel technologies and develop them and think two steps ahead.”
For Further Consideration
How do we create better knowledge and understanding? If we want this articulation to lead to better understanding and knowledge, how do we get there? How can we make that knowledge more accessible to journalists, researchers and policymakers? What can technology companies do? How can CNTI help distribute this knowledge?
2. WHETHER A PARTICULAR AI USE IS A BENEFIT OR HARM TO SOCIETY DEPENDS ON ITS CONTEXT AND DEGREE OF USE, MAKING SPECIFICITY VITAL TO EFFECTIVE POLICY.
Whether a particular use of AI is a benefit or a harm to society can depend on several factors, including the degree of use, the strength of protective actions and the subject matter surrounding the use. Effective policy must include specific and context-sensitive considerations. A prime example of this, discussed extensively by the group, is algorithmic transparency. (For more on this topic, see CNTI’s related Issue Primer.)
While there was general agreement on the importance of some level of transparency about how models are built and how newsrooms, journalists or others use AI – and, in most cases, agreement that more transparency is needed than currently exists – participants also discussed instances when transparency might be counterproductive. Is there a point at which the level of transparency carries more risk than value or causes more confusion than clarity – for instance, where “the level of transparency and detail … can actually undermine the security and safety of the models”? Determining where to draw that line is critical but difficult.
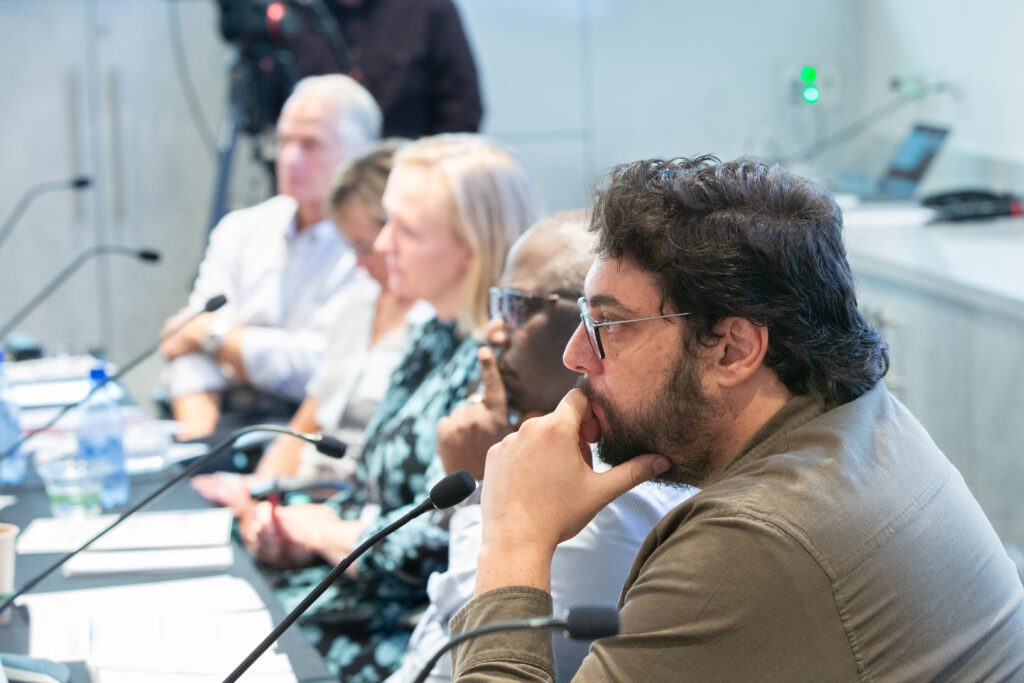
For example, optimal transparency around how AI is used in hiring processes or in making bail recommendations might differ from optimal transparency around the trillions of tokens that go into a LLM. The latter may not be as useful for unrestricted access to the public because of such varying abilities to evaluate this knowledge, and there could be a point at which the benefit of transparency is outweighed by the risk of malign actors abusing these systems. There seems to be clear value, though, in public transparency about whether content was fully AI-generated, was AI-generated with human involvement or came only from a human.
There were some different opinions about how to – or who should – determine how transparency extends to the broader public. One individual suggested “more transparency is better,” and then journalists can “make choices about how we describe all of this.” Participants agreed that we “have something to grapple with” and must work toward this together. These conversations call for “precision in the way we are discussing and analyzing different use cases so we ensure that policy that is created is specific to a given situation,” the challenge of doing so amid technology’s rapid evolution notwithstanding.
Another discussion surfaced about the benefits of AI that increased through advancements in LLMs, which AI policy may want to protect: transcription and translation. We “almost gloss over some of those [tools like transcription] because they’re such routinized things,” but “it is really the kind of scale of deployment that’s effective and interesting.” Similarly, translation has been used for a long time, but GAI has scaled it to create new opportunities for public use and benefit.
For Further Consideration
As we think about benefits of AI use, what are other AI practices that can be scaled up in ways that bring value to societies more broadly? What methods can be used to help determine how and when a benefit might become a harm, or vice versa?
3. EVEN WHEN POLICY IS GROUNDBREAKING, IT MUST ALSO TAKE INTO ACCOUNT HOW IT RELATES TO AND BUILDS ON PRIOR POLICY.
While it is important to focus on clear, specific definitions when constructing new regulations, the group recognized that policy development is a layered process, and it is necessary to consider it in the context of previously adopted policy. So while developing clear definitions around AI matters, existing policy will inevitably impact new policy.
Take, for example, anti-discrimination law. In many countries, discrimination is illegal regardless of how it occurs, including via AI. The specific definition of AI doesn’t really impact whether its use violates such laws. In a similar example, European Union and Canadian policies influence how AI gets trained and used in the context of data protection. Even if many of today’s AI systems did not exist when data protection laws were written, they still have an effect when it comes to AI. As one participant put it:
“Policy is often a layered process, where previously adopted laws matter. … It’s always a combination of things. … We are not starting totally from scratch, not legally and not procedurally.”
While there was clear agreement that definitions are important, at least one participant also asked: Have we reached a time where being clear about which principles should be protected is as important as the definitions themselves? Those principles can be articulated in ways that are technology-agnostic. Would this kind of approach help us create policy that can endure future technological developments? Or perhaps the answer is a nexus point of definitions and principles.
A core principle for CNTI, and for this convening, is a free and independent press, which is under intense pressure in many parts of the world. Could we look at the various ways in which uses of AI in journalism impact that principle and approach policy development with that lens?
Perhaps. But as other participants pointed out, that approach can be problematic if it is not done well, and definitions remain essential considerations. For example, a 2023 bill in Brazil proposed changing its Penal Code to include an article doubling the penalty for using AI to commit online fraud without actually defining AI – thus validating concerns about the impact of unclear definitions in legislative policy.
For Further Consideration
When it comes to AI use in journalism, what is the right balance between broad principles and specific definitions? Are there examples of successful regulatory structures that already exist, such as anti-discrimination policies, that can help us strike that balance?
4. ONE POLICY GOAL SHOULD BE TO ADDRESS DISPARITIES IN THE USES AND BENEFITS OF AI – AND PARTICULARLY GAI – AS A PUBLIC GOOD.
The importance of greater parity arose many times throughout the day. There are currently numerous global disparities in access to, use of and value of AI models. There was strong consensus that we need to work together on creating equality around AI as a public good, including the use of AI to help close gaps in news access. Inequalities work against the public good, which public policy is intended to protect. Use of and access to AI tools need to be democratized. How can public policy help that? Is this a case where organizational policy is as beneficial as — or perhaps more beneficial than — legislation?
Some specific areas of disparity discussed at the convening include:
The content in current AI models misses many people and many parts of the world. Certain parts of the world can’t realize the same potential of AI, especially GAI, because a substantial proportion of the world’s languages are not accounted for in LLMs (either because they do not have enough training data to create them or because tools aren’t well-constructed for them). While the practical effect of this disparity is easy to grasp, some less-considered impacts include reduced public trust in AI tools and their outputs.
GAI models often replicate existing social biases and inequities. Participants questioned the ability of many AI models to “reflect the full breadth of human expression,” noting examples ranging from the generation of photos of engineers as white men to the inclusion of QAnon conspiracy theories in LLM training data. Research supports this skepticism.
AI models, and the companies providing them, are largely exported from only a few countries. In many parts of the world, technological systems like GAI applications are largely imported from a small number of (often Western) companies. This means data used to build the models, as well as the models and tools, are imported from people and entities who likely do not understand the nuances of the information environment of the end users. This has led to greater public skepticism about — and even distrust toward — the implementation of these tools, both in general and in journalism specifically. One participant noted:
“There is a lot of aggravation that one can’t trust the technology because Africa is an importer of these AI tools.”
News is not a digital-first product in all parts of the world. Print, television and radio are still popular modes of news consumption in many countries. And there remain areas with much less internet accessthan others. So, as there is a push to digitization, “there’s an extent to which AI is still going to end up on the paper in some way, shape or form and we have to think about what that type of signaling may mean.” This raises the question, not yet given much consideration, of how AI use and policy translate to print.
Discussions should also consider ways policy can support use of AI as a means to help close gaps in news access. There are parts of global society with less access to independent, fact-based news – whether the result of financial downfalls (e.g., news deserts in the U.S.), government control or high-risk environments such as war zones. Could AI be helpful here? If so, how? “We need to look at ways that we can rebuild newsrooms and do so in a way where it’s not just the large players that survive,” expressed one participant, “but local newsrooms survive, that everyone can actually have access to information.”
Wealthier news publishers have a greater advantage when it comes to AI use and licensing. There are currently clear financial advantages for large, well-resourced — and often Western — national and international news outlets. As pointed out by one participant: “Licensing is one way to obtain information, but you need to have funds, you need to have means … those with the biggest purses can obtain data and can possibly benefit more from AI. … That is bad from a public policy perspective because it means we’re not actually democratizing access to model development.”
The varying relationships between governments and the press must be considered in policy discussions. In some contexts, policymakers simply may not value the principle of an independent press. In other contexts, such as in Caribbean nations, financial support via government ad spending is critical for the sustainability of media organizations, leading to a hesitance to create friction with their primary sources of funding. If governments do not trust media organizations’ adoption of AI tools, their actions can create economic problems for publishers or journalists.
For Further Consideration
What roles should legislative versus organizational policy play when it comes to addressing disparities in the use of AI as a public good?
5. BOTH INPUTS AND OUTPUTS, AT ALL STAGES OF BUILDING AND USING AI, NEED TO BE CONSIDERED THOROUGHLY IN POLICY DEVELOPMENT.
The last portion of the day was intended to be focused on copyright law, but many of the points raised during the discussion have broader policy implications. This section summarizes these points first, then makes note of considerations specific to copyright and AI in journalism.
A substantial and, as some participants noted, justifiable amount of attention and litigation has focused on the inputs of AI systems, particularly on what data models are being trained on, especially when it comes to news content. Less attention has been paid to the other end of the equation: these systems’ outputs. As one participant noted, there is only one ongoing case related to AI outputs. There was a general acknowledgement that policy and policy discussions must consider the totality of the system.
Two key issues emerged around AI outputs: ownership and liability. Both issues connect to copyright law and will likely be addressed in courts and public policy. One participant outlined three potential policy approaches:
- No AI-generated output is copyrighted and, thus, everything is in the public domain. This is the current policy approach in countries like the U.S.
- Some form of copyright of AI-generated output is recognized, as long as there is some degree of human intervention, and the human would receive the copyrights.
- AI-generated output receives short protections (e.g., 10 to 15 years) that could be registered to those who want to profit from transformative works.
Each option carries critical implications that are often lost in debates around AI inputs. Where is the cutoff point for AI-generated content? At what point in the editing process do we recognize content as having been transformed by AI and, therefore, no longer protected? Would this include the use of software, such as Photoshop, that have integrated AI tools? What does “human intervention” entail? And, for option three, what would be the duration of short-term protections (an option currently available in countries such as the United Kingdom)? Any policy, the group agreed, needs a clearly defined auditing process that includes evidence of steps taken in the content creation process.
Participants also discussed the lack of clarity around output protections when it comes to patterns of language that models learn from snippets of words (rather than agreed-upon protections for creative expression like written articles). This ambiguity underlies other critical questions around issues like disinformation, such as decisions about whether to pull content like QAnon conspiracy theories out of model training data.
Clarity around outputs should be added to (but not replace) conversations about inputs: decisions around what data AI models are, or should be, trained on. As one participant noted, “Sticking to an understanding of the inputs here is important when we describe this interplay between the models and journalism, because journalism very much needs to be rooted in fact-based reporting.” Some suggested that the inputs question is potentially the easier issue to solve outside the bounds of policymaking: “You just pay [for data].” Though, it is not clear where current copyright law would fall on this and, as noted earlier, can lead – and has led – to a winner-takes-most approach where technology companies and news publishers with the most money and resources have the most to gain.
This discussion also included a broader conversation about liability, both within the context of copyright policy and beyond it. Participants noted that one critical area in AI policymaking is about the various layers of responsibility for the application of expression via AI tools including the model itself, its API, and its application (e.g., ChatGPT):
“We need to think about what’s the framework to apportion responsibility and what responsibility lies at each level … so that you get the trust all the way up and down, because ultimately newsrooms want to be able to trust the technology they use and the end user wants to be able to trust the output or product from the newsroom.”
One element that came up at several points is the need for transparency from AI model developers in order to have more open discussions around how to apportion responsibility.
Spotlight on Copyright Law
OPPORTUNITIES FOR FURTHER ACTION
In addition to the areas for further consideration noted above, the convening introduced several potential opportunities for future work, including:
- The important remaining question of definitions of journalism in policy. While time limitations did not allow for a more thorough discussion of this question in this convening, participants noted a need to focus on definitions of journalism in policymaking alongside definitions of AI.
- Mapping the various elements of copyright and intellectual property protection and their impacts on journalism. While CNTI’s Issue Primer on copyright lays out the current state of copyright policy related to journalism, participants discussed the value of better understanding how exactly these laws apply to AI use in news. As one participant noted: “We should not only look at what makes sense for us in the here and now” but also “in contexts where different languages are spoken, where access is a huge problem, where skills are a huge problem, where disinformation has very different connotations … what can be the possible consequences for people who are more vulnerable, less empowered, in a different part of the world, and where the consequences can be way worse.”
- A need to take stock of AI practices (separate from guidelines), and establish a living repository of AI use cases related to journalism. CNTI developed a table of potential AI benefits and harms related to journalism (shown below) as a starting point for this discussion, though this was not an exhaustive list, and there is opportunity to develop it further into a digital resource. Participants also noted the need for an open database or repository of actual AI use cases (including their positive and negative impacts) that journalists and others creating and delivering fact-based news can add to. Some foundational work around this exists.
Realized & Potential Benefits
Realized & Potential Harms
Uses of AI models by journalists and newsrooms:
Efficiency in some tasks, enabling journalists to focus on more challenging work (e.g., transcription, translation, organization, summarization, data analysis, writing)
Loss of audience trust from errors or lack of transparency; IP infringement; journalistic loss of control
Easier mechanisms for content moderation
Potential for errors (false positives or negatives) and biases
Personalization and curation of news content for audiences
Implicit biases in methodological choices of models (language, social/cultural, technical)
Opportunities for innovation and open-access competition
Over-regulation that stifles innovation or benefits large, established news orgs and harms start-ups or freelancers
Accessible tools for new, local and smaller global newsrooms
Unequal global access and resources to invest in-house
Enhanced audience analytics/distribution (e.g., paywalls)
Unequal support for freelancers, creators, citizen journalists
Uses of AI models by third parties which may be used in reporting:
Capture of evidence in unsafe or inaccessible contexts (e.g., satellite imagery)
Use of this technology and data by those seeking to create disinformation, clickbait and scams
Aggregation and promotion of news content
Journalistic loss of control; reliance on third-party data
Delivery of fact-based news within & across borders, including in unsafe contexts (e.g., AI-enabledbots converting news content into accessible formats not blockable by governments)
Potential breaches of privacy regulations
Source or document verification through watermarks, etc.
Potential for errors; falsification by those seeking to create disinformation or confusion over facts
Automating time-consuming bureaucratic processes (e.g., FOIA requests)
Lack of human sensitivity; over-conservative approaches (e.g., over-redacting information)
Uses of news content by technology companies in AI models:
Corpus of easy-to-access information
Intellectual property or terms-of-service infringement; worsening financial strain on news publishers and journalists
Developing more comprehensive training data/AI models
Implicit biases in methodological choices of models
APPENDIX
List of Participants
Anna Bulakh Head of Ethics & Partnerships, Respeecher | Ben Petrosky Senior Policy Counsel, Google |
Garance Burke Global Investigative Journalist, The Associated Press | Kim Polese Chairman, CrowdSmart |
Craig Forman Managing General Partner, NextNews Ventures (CNTI Board) | Aimee Rinehart Local News & AI Program Manager, The Associated Press |
Richard Gingras Global VP of News, Google (CNTI Board) | Tom Rubin Chief of Intellectual Property & Content, OpenAI |
Andres Guadamuz Reader in Intellectual Property Law, University of Sussex | Marietje Schaake International Policy Director, Stanford Cyber Policy Center (CNTI Board) |
Dan’l Lewin President & CEO, Computer History Museum | Felix Simon Researcher, Oxford Internet Institute |
Megan Morrone Technology Editor, Axios | Krishna Sood Assistant General Counsel, Microsoft |
Dapo Olorunyomi Publisher, Premium Times | Sérgio Spagnuolo Founder/Executive Director, Núcleo Jornalismo |
Matt Perault Director, Center on Technology Policy | Scott Timcke Senior Research Associate, Research ICT Africa |
About This Convening
CNTI’s cross-industry convenings espouse evidence-based, thoughtful and challenging conversations about the issue at hand, with the goal of building trust and ongoing relationships along with some agreed-upon approaches to policy. To that end, this convening adhered to a slightly amended Chatham House Rule:
- Individuals are invited as leading thinkers from important parts of our digital news environment and as critical voices to finding feasible solutions. For the purposes of transparency, CNTI feels it is important to publicly list all attendees and affiliations present. Any reporting on the event, including CNTI’s reports summarizing key takeaways and next steps, can share information (including unattributed quotes) but cannot explicitly or implicitly identify who said what.
- CNTI does request the use of photo and video at convenings. Videography is intended to help with the summary report. Any public use of video clips with dialogue by CNTI or its co-hosts requires the explicit, advance consent of the subject.
- To maintain focus on the discussion at hand, we asked that there be no external posting during the event itself.
Participants were not asked to present prepared remarks; rather, this was a thoughtful guided discussion. To prepare, we asked that participants review CNTI’s Issue Primers on AI in journalism and modernizing copyright law.
Resources Shared by Participants
Participants at our convening event shared a number of helpful resources. Many of these resources are aimed at assisting local newsrooms. We present them in alphabetical order by organization/sponsor below.
The American Journalism Project (AJP), which has announced a new partnership with OpenAI, serves as a useful resource for local newsrooms, bolstering local news projects to ensure all communities in the U.S. have access to trusted information.
The Associated Press (AP) has launched, in addition to its Stylebook AI chapter, five new tools to improve workflow efficiencies in newsrooms with a focus on automation and transcription services. The AP also has assigned journalists on its global investigative team and beyond to cover artificial intelligence tools and their impacts on communities.
Another resource that organizations may find useful is CrowdSmart’s research on AI and conducting customer interviews, using AI to measure conversations and engage with human subjects, with the benefit of quantifying conversations in real time.
Google has developed Pinpoint, a digital tool for analyzing and transcribing documents, that organizes and facilitates document collection. The company has also launched its Data Commons resource which enables users to access publicly available data from around the world.
Microsoft’s Journalism Hub shares tools to promote sustainable approaches to local journalism and includes a partnership with NOTA, an journalist-founded AI startup aiming to streamline newsroom processes without replacing journalists. Meanwhile, Microsoft’s open-data campaign aims to address inequalities in access by developing datasets and technologies that make data sharing easier.
Finally, Respeecher, a novel startup founded in 2018, uses artificial intelligence to generate synthetic speech. The company has partnered with video game developers and motion picture studios to produce voices for characters – both fictional and real.
We appreciate all of our participants for sharing these resources with CNTI.
About CNTI
The Center for News, Technology & Innovation (CNTI), an independent global policy research center, seeks to encourage independent, sustainable media, maintain an open internet and foster informed public policy conversations. CNTI’s cross-industry convenings espouse evidence-based, thoughtful but challenging conversations about the issue at hand, with an eye toward feasible steps forward.
The Center for News, Technology & Innovation is a project of the Foundation for Technology, News & Public Affairs.
Acknowledgements
CNTI sincerely thanks the participants of this convening for their time and insights, and we are grateful to the Computer History Museum, the co-sponsor and host of this AI convening. Special thanks to Dan’l Lewin, Marguerite Gong Hancock and David Murphy for their support, and to Marietje Schaake for moderating such a productive discussion.
CNTI is generously supported by Craig Newmark Philanthropies, John D. and Catherine T. MacArthur Foundation, John S. and James L. Knight Foundation, the Lenfest Institute for Journalism and Google.